Unlocking the Future: The Importance of Training Data for Self-Driving Cars
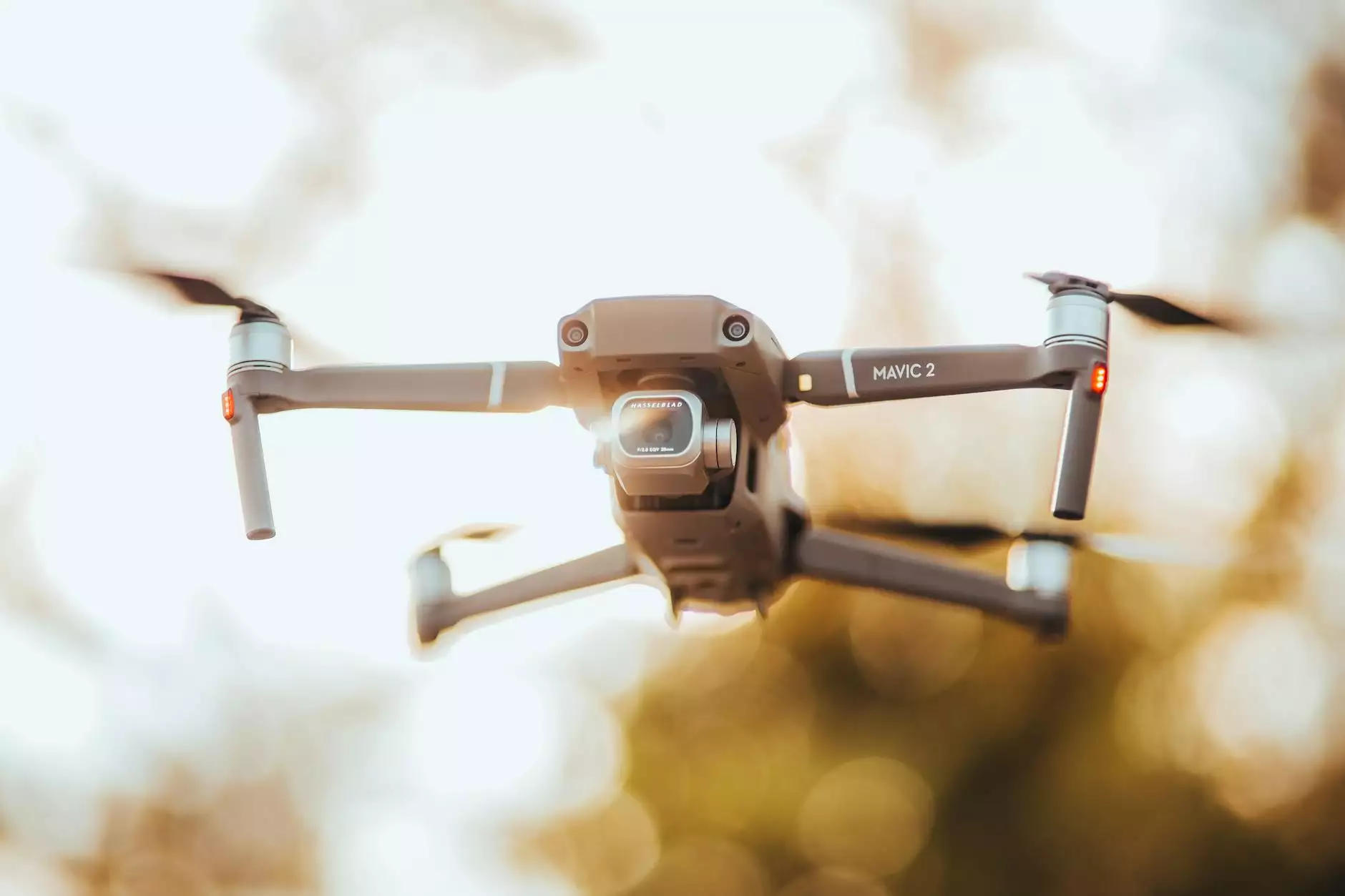
The advent of self-driving cars represents a monumental leap in technology that has the potential to redefine the landscape of transportation as we know it. Much of the incredible promise of autonomous vehicles is rooted in the quality and comprehensiveness of training data for self-driving cars. This data forms the backbone of machine learning algorithms that power these sophisticated systems, enabling them to navigate complex environments safely and efficiently.
Understanding Training Data for Self-Driving Cars
Training data comprises vast amounts of information gathered from various sources that are essential for teaching self-driving algorithms how to interpret the world around them. This data could include, but is not limited to, images, sensor readings, and real-time traffic conditions. The accuracy, diversity, and volume of this data directly influence the performance of autonomous vehicles.
Types of Training Data
When we talk about training data for self-driving cars, it is important to categorize the types of data involved:
- Visual Data: Images from cameras mounted on vehicles which help the system recognize objects like pedestrians, road signs, and other vehicles.
- Lidar Data: Utilizes light detection and ranging technology to create a three-dimensional map of the vehicle's surroundings.
- Radar Data: Helps in detecting objects and measuring their speed and distance, often used in adverse weather conditions.
- GPS Data: Critical for providing the vehicle with its geographical location.
- Vehicle Dynamics Data: Information on how a vehicle behaves under various conditions, crucial for understanding vehicle control.
The Role of Quality in Training Data
Not all training data is created equal. The effectiveness of training data for self-driving cars is determined by its quality. High-quality data sets are annotated accurately, represent a wide range of scenarios, and account for edge cases that vehicles may encounter in real-world situations.
The Importance of Diverse Scenarios
A self-driving car must be trained on a significant variety of scenarios to handle the unpredictability of real-world driving. This includes:
- Urban Driving: Navigating through busy city environments with pedestrians, cyclists, and complex intersections.
- Rural Driving: Handling unknown roads and recognizing wildlife or farm vehicles.
- Adverse Weather Conditions: Training data must include instances of rain, fog, snow, and ice to prepare vehicles for loss of traction and visibility.
- Night Time Driving: The data must cover how the vehicle performs in low light, recognizing objects and adjusting to various light sources like headlights and streetlights.
The Process of Data Collection
Collecting effective training data is no small feat. The process often involves several stages:
- Data Acquisition: Gathering raw data through sensors, cameras, and real-world driving experiences.
- Data Annotation: Enlisting the help of skilled annotators or automated tools to label data accurately for machine learning models.
- Data Management: Storing and organizing data efficiently to facilitate easy access and processing.
- Data Augmentation: Enhancing existing data sets by creating altered versions to simulate various conditions and environments.
Machine Learning and Its Dependencies
At the core of self-driving technology is machine learning, which relies heavily on the quality of training data. Different algorithms require different types of data for training:
- Supervised Learning: Involves training models on labeled datasets, i.e., training data where inputs are paired with correct outputs.
- Unsupervised Learning: Utilizes data without specific outputs, focusing on identifying patterns and correlations in the data.
- Reinforcement Learning: A trial-and-error approach where agents learn to make decisions by receiving feedback from actions performed.
Challenges and Solutions in Data Collection
While the potential of training data for self-driving cars is immense, there are several challenges that companies face in data collection and utilization:
Challenges
- Inaccurate data can lead to faulty algorithms, resulting in unsafe vehicle behavior.
- Data privacy concerns may arise when collecting information in public spaces.
- Balancing the need for a vast dataset with the limitations of time and resources.
- Ensuring data diversity to account for various geographical contexts and driving conditions.
Solutions
In addressing these challenges, companies can adopt several strategies:
- Utilizing Simulation Tools: By employing advanced simulation software, companies can generate vast amounts of virtual data that cover numerous scenarios without the constraints of real-world data collection.
- Collaborating with Other Entities: Partnering with research institutions or sharing data among competing companies can enhance data quality and diversity.
- Implementing Robust Data Validation: Ensuring data integrity through rigorous validation processes to filter out inaccurate or low-quality data.
The Future of Training Data for Self-Driving Cars
The future of training data for self-driving cars holds tremendous promise. As technology evolves, we can expect:
- Increased Use of AI and Machine Learning: Algorithms will become more sophisticated, enabling them to learn from fewer examples and adapt to new environments quickly.
- Enhanced Data Privacy Measures: Companies will need to take significant steps to ensure compliance with data protection regulations while still gathering necessary data.
- Greater Integration of Real-Time Data: Future vehicles will likely utilize real-time data from surrounding cars and infrastructure, enhancing situational awareness and decision-making capabilities.
How Keymakr.com is Leading the Way
As a frontrunner in the realm of software development, keymakr.com is at the forefront of revolutionary innovations concerning training data for self-driving cars. With our commitment to quality, we ensure that our clients receive comprehensive and meticulously curated datasets that empower autonomous vehicles to operate safely and efficiently.
Our Approach
At Keymakr, we employ cutting-edge techniques and technologies in the data collection and annotation process, delivering:
- High-quality annotated datasets tailored specifically for the unique requirements of self-driving technology.
- Adaptive data solutions that evolve with the rapidly changing landscape of transportation and technology.
- Dedicated AI models and platforms that help process vast amounts of information quickly and accurately.
Conclusion: A Bright Future Ahead
The journey towards fully autonomous vehicles is a multi-faceted endeavor, deeply reliant on the training data for self-driving cars. As technology progresses, the methodologies for collecting and utilizing data will advance, ensuring that safety, efficiency, and innovation remain at the forefront of the industry. At Keymakr, we are excited to help shape the future of autonomous driving through our commitment to high-quality software development and data solutions.
training data for self driving cars